HYPER-PARAMETER TUNING PADA XGBOOST UNTUK PREDIKSI KEBERLANGSUNGAN HIDUP PASIEN GAGAL JANTUNG
Abstract
Prediksi keberlangsungan hidup pasien gagal jantung telah dilakukan pada penelitian untuk mencari tahu tentang kinerja, akurasi, presisi dan performa dari model prediksi ataupun metode yang digunakan dalam penelitian, dengan menggunakan dataset heart failure clinical records. Namun dataset ini memiliki permasalahan yaitu bersifat tidak seimbang yang dapat menurunkan kinerja model prediksi karena cenderung menghasilkan prediksi kelas mayoritas. Pada penelitian ini menggunakan pendekatan level algoritma untuk mengatasi ketidakseimbangan kelas yaitu teknik boosting dengan metode XGBoost lalu digabungkan dengan metode hyper-parameter tuning agar kinerja yang dihasilkan menjadi lebih baik. Selanjutnya model dilatih dengan dataset dan dibandingkan dengan metode lain, hasilnya menunjukkan bahwa XGBoost dengan tree parzen estimator hyper-parameter tuning mengungguli model lain dan mencapai nilai AUC sebesar 0.94, untuk model XGBoost dengan Random Search memperoleh nilai AUC sebesar 0.933, kemudian untuk model XGBoost dengan Grid Search memperoleh nilai AUC sebesar 0.922, lalu untuk model XGBoost tanpa hyper-parameter tuning memperoleh nilai AUC sebesar 0.904.
Keywords: Ketidakseimbangan Kelas, XGBoost, Konfigurasi Hyper-parameter, Gagal Jantung
Full Text:
PDFReferences
Rozie, F., & Trias Pontia, F. 2016. “Rancang Bangun Alat Monitoring Jumlah Denyut Nadi Jantung Berbasis Android”. Jurusan Teknik Elektro Fakultas Teknik Universitas Tanjungpura.
Chicco, D., & Jurman, G. 2020. “Machine learning can predict survival of patients with heart failure from serum creatinine and ejection fraction alone”. BMC Medical Informatics and Decision Making, 20(1). https://doi.org/10.1186/s12911-020-1023-5
Wahono, R. S., & Suryana, N. 2013. “Combining particle swarm optimization based feature selection and bagging technique for software defect prediction”. International Journal of Software Engineering and Its Applications, 7(5), 153–166. https://doi.org/10.14257/ijseia.2013.7.5.16
Chen, T., & Guestrin, C. 2016. “XGBoost: A scalable tree boosting system”. Proceedings of the ACM SIGKDD International Conference on Knowledge Discovery and Data Mining, 13-17-August-2016, 785–794. https://doi.org/10.1145/2939672.2939785
Tama, B. A., Nkenyereye, L., Islam, S. M. R., & Kwak, K. S. 2020. “An enhanced anomaly detection in web traffic using a stack of classifier ensemble”. IEEE Access, 8, 24120–24134. https://doi.org/10.1109/ACCESS.2020.2969428
Dong, H., He, D., & Wang, F. 2020. “SMOTE-XGBoost using Tree Parzen Estimator optimization for copper flotation method classification”. Powder Technology, 375, 174–181. https://doi.org/10.1016/j.powtec.2020.07.065
Bergstra, J., Bardenet, R., Bangio, Y., & Kégl, B. 2011. “Algorithms for Hyper-Parameter Optimization”. Advances in Neural Information Processing Systems 24 (NIPS 2011), 24.
Huang, Q., Mao, J., & Liu, Y. 2012. “An Improved Grid Search Algorithm of SVR Parameters Optimization”. IEEE.
Priscilla, C. V., & Prabha, D. P. 2020. “Influence of optimizing xgboost to handle class imbalance in credit card fraud detection”. Proceedings of the 3rd International Conference on Smart Systems and Inventive Technology, ICSSIT 2020, 1309–1315. https://doi.org/10.1109/ICSSIT48917.2020.9214206
Wang, Y., & Sherry Ni, X. 2019. “AN XGBOOST RISK MODEL VIA FEATURE SELECTION AND BAYESIAN HYPER-PARAMETER OPTIMIZATION”. International Journal of Database Management Systems, 11(01), 01–17. https://doi.org/10.5121/ijdms.2019.11101
Ogunleye, A., & Wang, Q. G. 2020. “XGBoost Model for Chronic Kidney Disease Diagnosis”s. IEEE/ACM Transactions on Computational Biology and Bioinformatics, 17(6), 2131–2140. https://doi.org/10.1109/TCBB.2019.2911071
Gertz, M., Große-Butenuth, K., Junge, W., Maassen-Francke, B., Renner, C., Sparenberg, H., & Krieter, J. 2020. “Using the XGBoost algorithm to classify neck and leg activity sensor data using on-farm health recordings for locomotor-associated diseases”. Computers and Electronics in Agriculture, 173. https://doi.org/10.1016/j.compag.2020.105404
DOI: http://dx.doi.org/10.20527/klik.v9i2.484
Copyright (c) 2022 KLIK - KUMPULAN JURNAL ILMU KOMPUTER

This work is licensed under a Creative Commons Attribution 4.0 International License.



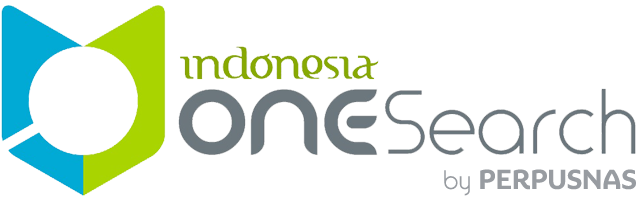
This work is licensed under a Creative Commons Attribution 4.0 International License. View My Stats