Sistem Rekognisi Jenis Kendaraan Dengan Analisis Video Menggunakan Metode Yolov4
Abstract
Full Text:
PDFReferences
Samsir and J. H. P. Sitorus, “Perancangan Sistem Monitoring Lokasi Kendaraan Menggunakan GPS U-Blox Berbasis Android,” J. Bisantara Inform., vol. 5, no. 1, pp. 1–10, 2021.
M. Rusmin, “Analisis Kinerja Di Simpang Empat Tak Sebidang Kota Makassar Berbasis Mikrosimulasi,” vol. l, 2018, [Online]. Available: http://dx.doi.org/10.31227/osf.io/sbv73
A. A. B, A. Amin, and M. W. Kasrani, “Penerapan Metode Yolo Object Detection V1 Terhadap Proses Pendeteksian Jenis Kendaraan Di Parkiran,” J. Tek. Elektro Uniba (JTE UNIBA), vol. 6, no. 1, pp. 194–199, 2021, doi: 10.36277/jteuniba.v6i1.130.
N. Hanum Harani, C. Prianto, and M. Hasanah, “Deteksi Objek Dan Pengenalan Karakter Plat Nomor Kendaraan Indonesia Menggunakan Metode Convolutional Neural Network (CNN) Berbasis Python,” J. Tek. Inform., vol. 11, no. 3, pp. 47–53, 2019.
F. Rachmawati and D. Widhyaestoeti, “Deteksi Jumlah Kendaraan di Jalur SSA Kota Bogor Menggunakan Algoritma Deep Learning YOLO,” pp. 360–370.
M. H. Yusfina, C. Setianingsih, and R. Astuti, “Deteksi Pelanggaran Parkir Pada Bahu Jalan Tol Dengan Intelligent Transportation System Menggunakan Algoritma Yolo ( Parking Violation Detection On The Roadside Of Toll Roads With Intelligent Transportation System Using Yolo Algorithm ),” e-Proceeding Eng., vol. 9, no. 3, pp. 1064–1069, 2022.
R. M. Taufiq, Sunanto, Y. Rizki, and M. R. A. Pratama, “Simulasi Deteksi Golongan Kendaraan pada Gerbang Tol Menggunakan YOLOv4,” J. CoSciTech (Computer Sci. Inf. Technol., vol. 3, no. 2, pp. 199–206, 2022, doi: 10.37859/coscitech.v3i2.3928.
J. Zhao et al., “Improved Vision-Based Vehicle Detection and Classification by Optimized YOLOv4,” IEEE Access, vol. 10, no. i, pp. 8590–8603, 2022, doi: 10.1109/ACCESS.2022.3143365.
M. I. HermaTelkomwan et al., “Kendaraan Menggunakan Metode Yolo Traffic Light Control Based on Vehicle Density Using the Yolo,” vol. 8, no. 1, pp. 198–205, 2021.
Y. Zhang, Z. Guo, J. Wu, Y. Tian, H. Tang, and X. Guo, “Real-Time Vehicle Detection Based on Improved YOLO v5,” Sustain., vol. 14, no. 19, 2022, doi: 10.3390/su141912274.
DOI: http://dx.doi.org/10.20527/klik.v11i1.625
Copyright (c) 2024 KLIK - KUMPULAN JURNAL ILMU KOMPUTER

This work is licensed under a Creative Commons Attribution 4.0 International License.



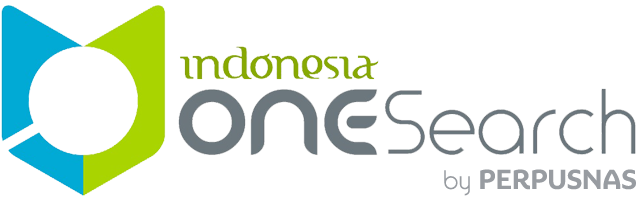
This work is licensed under a Creative Commons Attribution 4.0 International License. View My Stats